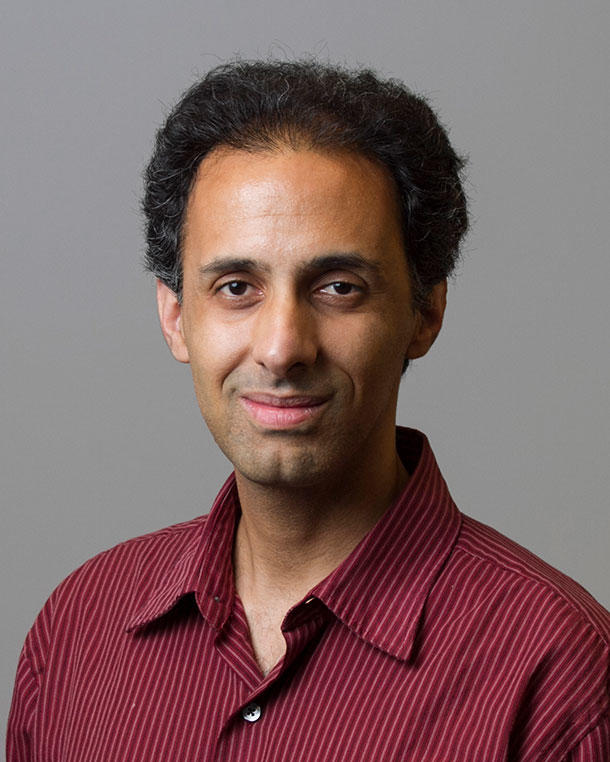
Hormuzd A. Katki, Ph.D.
NCI Shady Grove | Room 7E592
Biography
Hormuzd A. Katki received a B.S. in math from the University of Chicago, Illinois, and an M.S. in statistics from Carnegie-Mellon University, Pittsburg, PA. He received a Ph.D. in biostatistics from Johns Hopkins University, Baltimore, MD, in 2006, where he received the Margaret Merrell Award for research by a biostatistics doctoral student. Dr. Katki joined NCI in 1999, became a principal investigator in 2009, and was appointed senior investigator upon receiving NIH scientific tenure in 2015.
Research Interests
Dr. Katki’s research focuses on understanding how epidemiologic findings could be used for cancer screening and prevention. He is particularly interested in developing individualized risk-based approaches to cancer screening. His methodologic research focuses on estimating individual absolute risk, strategies for risk-based screening and management, and metrics for evaluating risk models and biomarkers. Dr. Katki mentors both statisticians interested in cancer research and epidemiologists who want to use state-of-the-art quantitative and computational methods.
Lung Cancer Screening
In spite of the definitive National Lung Screening Trial (NLST) and U.S. Preventive Services Task Force (USPSTF) guidelines recommending screening, CT lung-cancer screening is still not widespread. This is partly due to the inefficiency of screening. To make screening more efficient, Dr. Katki conducts research on the use of risk calculations to better identify those who would benefit the most from lung screening and to propose risk-based management options during the course of screening.
- Dr. Katki developed validated individualized risk models for lung cancer incidence (LCRAT: Lung Cancer Risk Assessment Tool) and mortality (LCDRAT: Lung Cancer Death Risk Assessment Tool). He and Dr. Li Cheung developed the LYFS-CT (Life-Years From Screening-CT) model for individualized life-gained from screening, which incorporates life-expectancy. Using these models to select ever-smokers at highest risk should improve screening effectiveness and efficiency, and identifies more high-benefit racial/ethnic minorities versus current USPSTF guidelines. To empower doctors and patients with risk information needed to decide about undergoing screening, Dr. Katki is collaborating with Dr. William Klein (DCCPS) to improve the NCI lung cancer screening risk tool, the Risk-based NLST Outcomes Tool (RNOT). In addition, Dr. Katki’s models are the computational engine for a prediction-based online lung screening tool and the EPIC EHR clinical decision support intervention.
- The R package lcmodels estimates risk from ten published lung cancer prediction models: LCRAT, LCDRAT, LYFS-CT, Bach, PLCOM2012, Spitz, Hoggart, LLP, LLPi, and Pittsburgh. The R package lcrisks provides the risk calculators that are used by RNOT and screenlc.com.
- Dr. Katki and Dr. Hilary Robbins developed a Markov model, LCRAT+CT, that updates individual lung cancer risk with CT image findings (either radiologic features or AI algorithm score) during the course of screening. This model may be useful to extend screening intervals for those at sufficiently low risk of developing lung cancer.
Improving External Validity of Epidemiologic Analyses and Risk Models
Participants in cohort studies are often healthier than the general population and underrepresent minority populations. BB investigators, including Drs. Katki, Barry Graubard, and Lingxiao Wang have been developing methods using survey data to create "pseudoweights" for a cohort so that analyses are more population representative. This facilitates estimating national prevalences of risk factors or distributions of disease risks. In addition, BB investigators have recently developed methodology for individualized absolute risk models that are nationally calibrated automatically, by incorporating survey data and summary statistics from national registries. The approach requires a correct propensity model to generate pseudoweights for the cohort, but (1) does not require transportability assumptions between data sources (because pseudoweights and post-stratification improve population representativeness) and (2) provides design-consistent inference for absolute risks, regardless of whether the chosen risk model is the true data-generating mechanism.
Enhancing Research on Underserved Populations
Prediction models have been criticized for not ensuring fairness. Drs. Katki and Rebecca Landy (CGB) showed that USPSTF lung screening eligibility criteria (ages 50-80, ≥20 pack-years, ≤15 quit-years) could induce racial/ethnic disparities, in the sense that the fractions of savable lives and gainable life-years are substantially larger for white Americans than any minority. However, augmenting USPSTF criteria to also include people at high benefit, as chosen by the LYFS-CT model, might reduce or eliminate the disparity between white Americans and African Americans. Ongoing work includes examining algorithmic fairness of use of prediction models in screening and developing a nationally representative cohort of racial/ethnic minorities.
Screening with Multicancer Early Detection (MCED) Tests
MCED tests could facilitate cancer screening at multiple organ sites with a single blood test. Dr. Katki is helping design prospective MCED studies and leads a team to research innovative methodologic issues for potential screening trial designs. Dr. Katki is also interested in projecting the benefits and harms of such screening.
Risk Models for Epidemiology
Dr. Katki is interested in developing models for individualized risk estimation, for example:
- Drs. Katki and Cheung developed risk models for screening data, where some disease is already present at baseline (left-censored), some disease occurs between consecutive visits (interval censored), and some disease is unknown if it was prevalent or incident. These models, the logistic-Weibull and logistic-Cox models, can be accessed as part of R package PImixture. The models allow sampling weights.
- Dr. Katki has helped develop methods and software for calculating absolute risk for case-cohort studies, or case-control studies nested within cohorts (also known as “two-phase sampling”), which is in the R package NestedCohort.
- Dr. Katki has proposed a hybrid risk regression model called “LEXPIT” that allows for both additive and multiplicative effects in logistic regression, and allows sampling weights. LEXPIT is in the R package blm.
- Drs. Katki and Graubard are conducting research on improving the external validity of epidemiologic cohort analyses by including data from nationally representative surveys.
Metrics for Evaluating Diagnostic Tests and Risk Prediction Models
Dr. Katki is interested in all aspects of evaluating the potential of new biomarkers for clinical use.
- In particular, he has done research to quantify risk stratification, the ability of a test or model to separate those at high risk from those at low risk. His metric—mean risk stratification (MRS)—is the average change in a person’s risk that is revealed by using a risk model or test. MRS better compares tests across populations with different disease prevalence by interpreting the area under the ROC curve (AUC) in the context of prevalence. He has used MRS to compare the risk stratification from cervical screening tests and risk models to identify who in a family carries a variation in BRCA1/2. The MRS web tool is part of the Biomarker Tools Suite.
However, MRS is not meant to account for the benefits, harms, and costs of tests and interventions. Dr. Katki developed a simple framework to calculate the incremental net benefit for a single-time screen as a function of costs (for tests and treatments) and effectiveness (life-years gained), providing simple expressions for the optimal cost-effective risk threshold and the monetary value of life-years gained associated with a threshold. Unlike MRS or decision curve analysis, this framework can identify optimal risk-thresholds and facilitates sensitivity analyses to cost/benefit parameters. - Dr. Katki also has developed methods for calculating diagnostic accuracy and agreement statistics under verification bias, when one test is conducted on only a sub-sample of specimens, in R package CompareTests.
Cervical Cancer Screening and HPV-related Cancers
- Dr. Katki led a team that calculated cervical cancer risks, using the logistic-Weibull model, using data on 1.4 million women at Kaiser Permanente Northern California (KPNC). These data enabled the development of clinical practice guidelines to ensure “equal management of women at equal risk of cancer.” The resulting 2012 ASCCP Guidelines and the eight reports with the supporting data were published in a 2013 supplement of the Journal of Lower Genital Tract Disease.
- He developed the “Risk Bar” for the risk-based App for the Consensus Guidelines for the Management of Abnormal Cervical Cancer Screening Tests and Cancer Precursors, based on patient’s history of HPV, Pap test, and biopsy results.
- Dr. Katki collaborates with Dr. Anil Chaturvedi on oral HPV and oropharyngeal cancer, conducting research on natural history with an eye towards future prevention.
Population-Based Mutation Screening
Dr. Katki is developing risk-based approaches to help propose screening programs for variants in high-risk genes, such as for BRCA1 and BRCA2.
Press Contacts
To request an interview with NCI researchers, contact the NCI Office of Media Relations.
ncipressofficers@mail.nih.gov | 240-760-6600