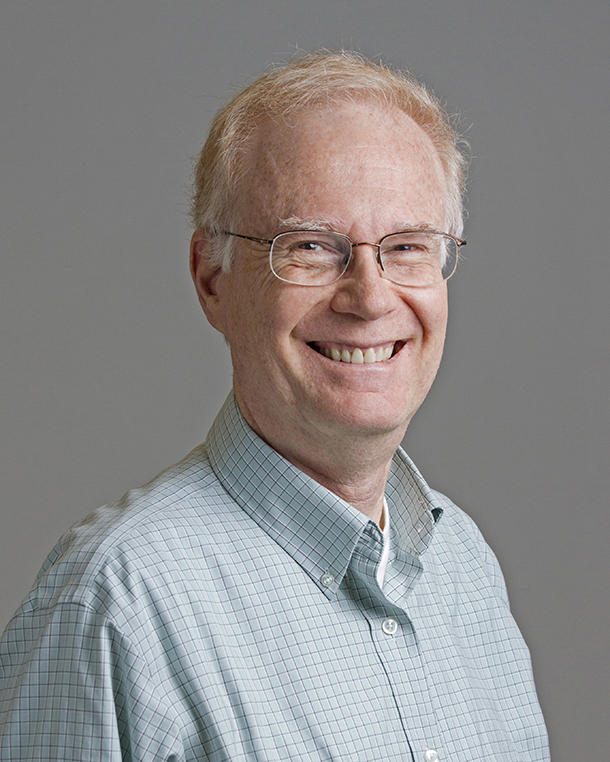
Biography
Dr. Graubard received a Ph.D. in mathematics from the University of Maryland in 1991. He began his career as a mathematical statistician at the National Center for Health Statistics in 1977 and held research positions at the Alcohol Drug Abuse and Mental Health Administration and the National Institute of Child Health and Human Development. Dr. Graubard joined the National Cancer Institute in 1990. In 2020, he was recognized with the ASA Mentoring Award. At the beginning of 2024, Dr. Graubard retired from government service and continues to support DCEG as a Scientist Emeritus.
Throughout his career, Dr. Graubard focused on developing new statistical methodologies at the interface between biostatistics and survey sampling with a particular emphasis on cancer epidemiology, making fundamental contributions to the use of national population-based surveys to improve the representativeness of cohort, case-control, nested case-control, and cross-sectional studies. In 1999, together with Dr. Edward Korn, a statistician in the NCI Division of Cancer Diagnosis and Treatment, he published Analysis of Health Surveys, which remains the definitive textbook in this field. In summary, a large part of Dr. Graubard's research has involved the integration of survey methods to develop statistical methods for efficiently using complex sample designs in cancer surveillance and epidemiology.
Dr. Graubard received the American Statistical Association (ASA) and Biometric Society Snedecor Award for Applied Statistical Research in 1990, and he is a Fellow of the American Statistical Association and of the Statistics Section of the American Association for the Advancement of Science.