Dr. Wenyi Wang - Deciphering Cancer Cell Evolution and Ecology
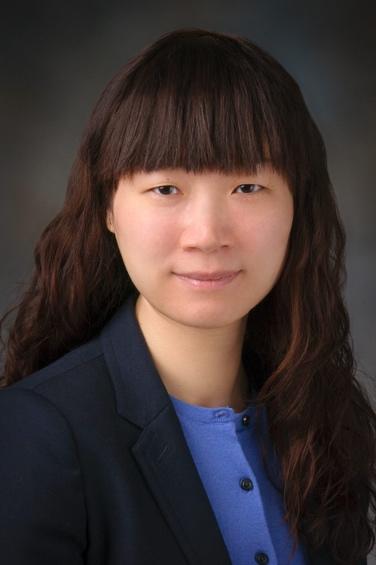
Dr. Wenyi Wang
February 23, 2023 | 10:30 AM – 11:30 AM
Zoom
Speaker
Wenyi Wang, Ph.D., Professor
Department of Bioinformatics and Computational Biology and Department of Biostatistics
The University of Texas
MD Anderson Cancer Center
Title
Deciphering Cancer Cell Evolution and Ecology
Join the Meeting
Abstract
In most cancers, the heterogeneity of cell composition within and between tumor samples is mirrored in complex variations at the molecular level. This molecular complexity includes both transcriptional variation (i.e., ecology) and genomic complexity (i.e., evolution) as tumors continually evolve and acquire new mutations. Therefore, to further our understanding of tumor evolution, it is essential to study the evolutionary dynamics between cancer genomes and transcriptomes. However, due to the complex interplay between cancer cells and their environments, these dynamics are still poorly understood, presenting a major bottleneck for the advancement of clinical management and treatment of cancer patients. Building on previous work in DeMixT for transcriptomic deconvolution, we have developed DeMix.SC, a new approach to deconvolve bulk RNA sequencing data using single-cell reference profiles. DeMix.SC is unique in taking advantage of a benchmark dataset to estimate systematic platform errors and specifically account for them during deconvolution. We will use a retina dataset to demonstrate improved deconvolution performance because of such adjustment. Furthermore, building on previous work in MuSE for subclonal somatic mutation calling, we have developed Clonal structure identification through pair-wise Penalization (CliP) to quantify the genomic dynamics. We found CliP is robust and accurate when applied to both whole genome (PCAWG, n = 1,993) and whole exome sequencing (TCGA, n = 7,711) data spanning >30 cancer types. The reconstructed tumor subclonality associates significantly with clinical subtypes and prognosis. In summary, quantitative measurement of heterogeneity in tissue samples at scale using multi-omics data remains an open and potentially impactful problem for the treatment of cancer and other diseases.
Host
Li C. Cheung, Ph.D., Earl Stadtman investigator
Biostatistics Branch