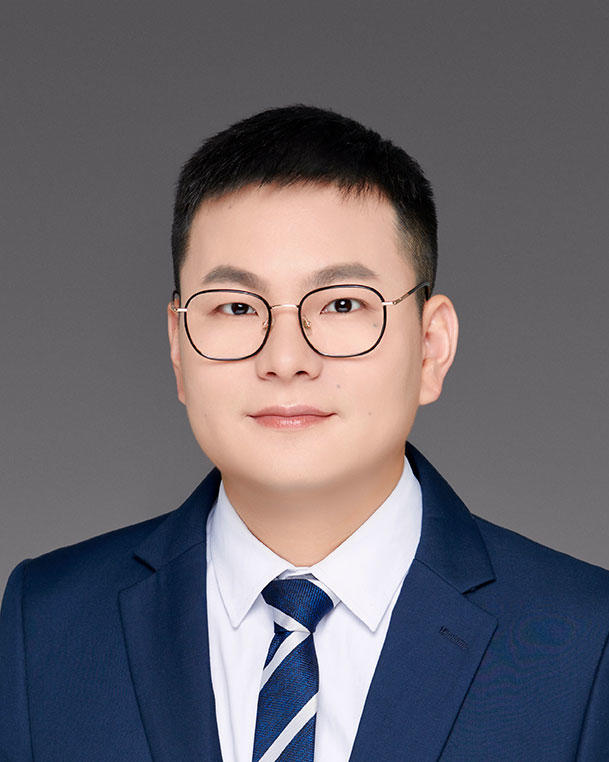
Tongwu Zhang, Ph.D.
NCI Shady Grove | Room 7E594
Biography
Dr. Tongwu Zhang received his Ph.D. in bioinformatics from Zhejiang University, China, in June 2012. He conducted part of his doctoral research at the Beijing Institute of Genomics, Chinese Academy of Science, where he worked on whole-genome sequencing and assembly based on different next-generation sequencing technologies. Before joining NCI, he was a visiting scholar at the King Abdulaziz City for Science and Technology, Kingdom of Saudi Arabia. Dr. Zhang joined the Laboratory of Translational Genomics (LTG) as a visiting postdoctoral fellow in July 2012, under the mentorship of Kevin M. Brown, Ph.D. He became a staff scientist and worked with Maria Teresa Landi, M.D., Ph.D., senior investigator, in the Integrative Tumor Epidemiology Branch (ITEB) in October 2017. In 2023, Dr. Zhang was appointed as an Earl Stadtman tenure-track investigator in the Biostatistics Branch (BB).
Dr. Zhang has received numerous awards for his work, including an NCI Director’s Award, NCI Director’s Innovation Award, CCR-DCEG FLEX Award, and several awards from DCEG.
Research Interests
Dr. Zhang's research focuses on characterizing tumor heterogeneity and evolution in relation to specific genetic backgrounds and etiological factors. As an investigator in BB, he develops novel analytical approaches to dissect complex genomic features and investigates the germline-somatic etiological links in large datasets with epidemiological and clinical information across diverse tumor types and populations.
Genomic Analyses for Sherlock-Lung
Lung cancer in never-smokers (LCINS) accounts for 10%-25% of lung cancer cases and ranks among the most common causes of cancer mortality. The Sherlock-Lung study aims to trace lung cancer etiology in never-smokers through a comprehensive multi-omics approach, including conducting whole genome sequencing, whole transcriptome, and genome-wide methylation for tumors and surrounding lung tissue. Dr. Zhang leads genomic analyses and develops new bioinformatic pipelines to 1) characterize the genomic, epigenomic, and transcriptomic landscape of LCINS; 2) identify the exogenous and endogenous mutational processes involved in lung tumorigenesis in LCINS; and 3) study the tumor evolutionary history of LCINS.
Exploring Complex Cancer Genomic Features
In-depth genomic analyses of tumor profiling data can elucidate etiological factors and carcinogenic processes, which in turn can improve cancer prevention and treatment strategies. Leveraging data from large-scale genomic studies of different cancer types, Dr. Zhang focuses on advancing cancer genomics by developing novel analytical approaches to study complex genomic features, such as extrachromosomal DNA (ecDNA) and mutational signatures. Studying these genomic features will help us to better understand tumor etiology and mechanisms of carcinogenesis. To elucidate germline effects on the somatic mutational landscape of human cancer, he performs integrative analysis to unravel the intricate interplay of germline variants and these identified somatic features. Over time, Dr. Zhang plans to extend these approaches to analyze haplotype-resolved genomic data based on personalized population-specific reference genomes.
Bioinformatics Tools Development for Cancer Genomics and Genetic Study
Dr. Zhang is the creator of a web-based tool, ezQTL, used for interactive visualization and colocalization of quantitative trait loci (QTL) and genome-wide association studies (GWAS). For his work in cancer genomics, he also created mSigPortal, an integrative mutational signature portal designed to visualize, analyze, and explore the mutational signature data from public genomic studies and/or the user's input. mSigPortal will greatly benefit studies investigating mutagenetic processes involved in tumorigenesis. Dr. Zhang also is developing PurityNGS, a software that can be used to visualize and estimate tumor purity, ploidy, and clonal architecture by integrating somatic copy number alteration, single nucleotide variants, and cancer cell fraction.
Press Contacts
To request an interview with NCI researchers, contact the NCI Office of Media Relations.
ncipressofficers@mail.nih.gov | 240-760-6600